Today, sharing economy marketplaces miss out on opportunities to improve their risk management performance because they focus too much on user-level verifications, by just running background checks for example. While this is an important part of the equation, the total risk of a transaction is also related to entities such as the asset, the location, the context of the transaction, communications, etc. Therefore, a world-class risk assessment should go beyond just verifying users.
We’re going to present a framework illustrated with real examples that will help marketplaces improve their performance by assessing risks holistically.
The “Growth vs Risk” Dilemma
Adjusting the risk exposure of your marketplace is an ever-going exercise. On one side of the spectrum, the no-risk scenario is pretty simple: just don’t let any transaction go through your marketplace. On the other side, if you accept all transactions you’re taking full advantage of your growth potential but most likely going straight into the wall as your risk exposure is maximal.
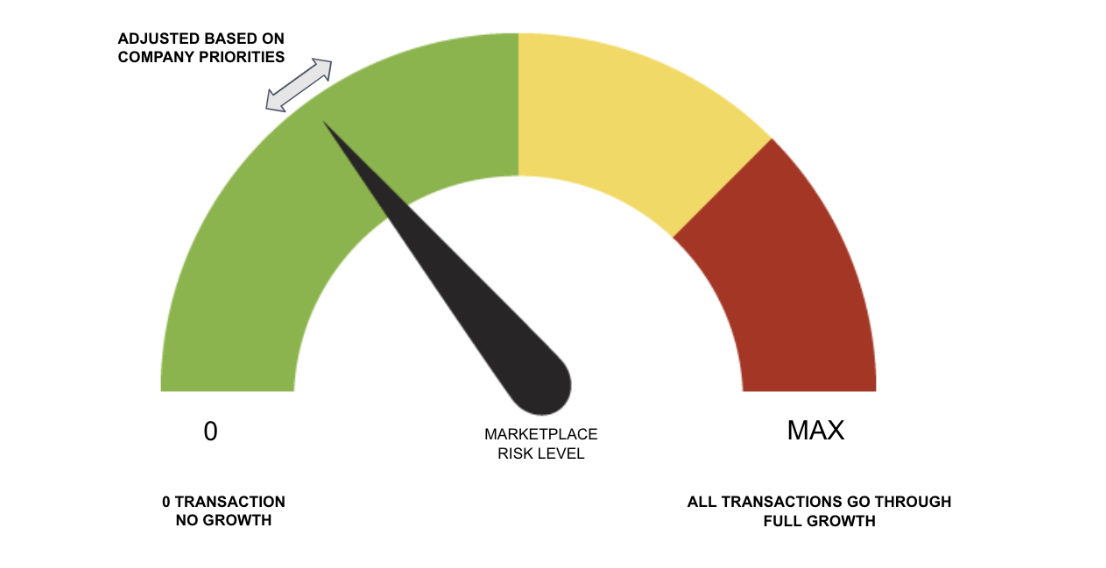
The answer is of course in the middle. Managing risk in a high growth environment requires constantly adapting to the new typology of transactions you’re seeing, using what you’ve learnt from the past and adapting to the latest trends and threats.
Overview of Risks for Most Sharing Economy Marketplaces
Risk at most sharing economy marketplaces is multi-faceted — these risks go from fraud, to incidentals, property damage, liability or even bad debt. In order to manage each of them, marketplaces need to put in place a specific financial structure: either transfer them to an insurer or self insure.
Below is an overview of the different types of risks we’ve identified working with several sharing economy marketplaces and how these marketplaces usually manage them.
Is your marketplace facing other types of risks? Let us know, we’re happy to chat about it and help you find solutions to manage them. But remember, it’s very important that every occurrence of the risk you’re trying to mitigate is properly documented: “if we can measure it, we can improve it”!
Framework for Optimal Risk Modeling
When it comes to modeling in general, a holistic approach considering all the potential variables will increase the precision and the accuracy of your models. Risk modeling just follows the rule. When working on predicting risk occurrences of a sharing economy marketplace, you should not only consider the users but also the assets and the context in which the transaction is happening. Below is an example of the variables you can consider.
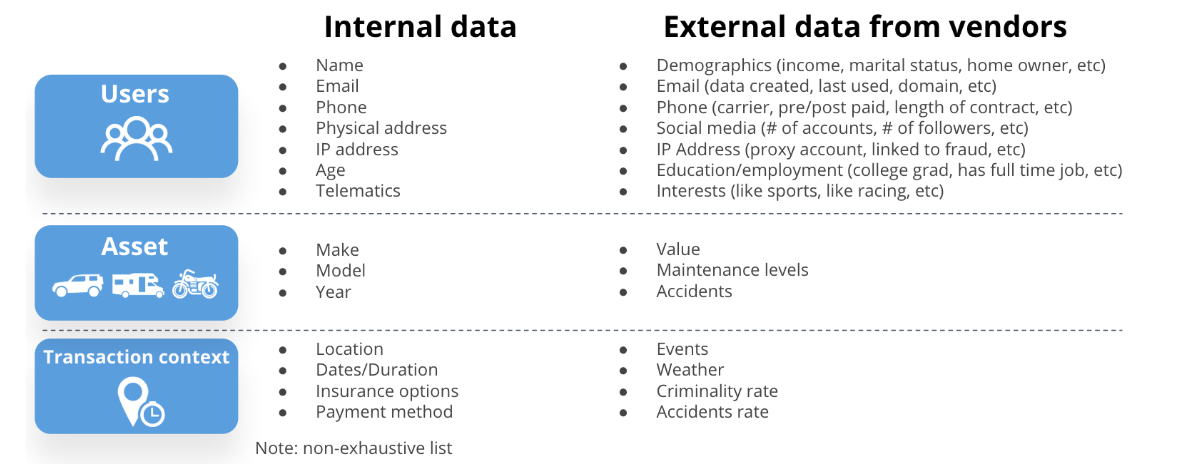
For users, assets and transactions, you can obviously leverage your internal data but it’s also important to think outside-the-box and consider data and variables that come from 3rd party sources.
Joining all the user, asset and transaction variables with the risk occurrences dataset will let you build the training set required to train and calibrate machine learning models. The outcomes of machine learning models can be then transformed into scores.
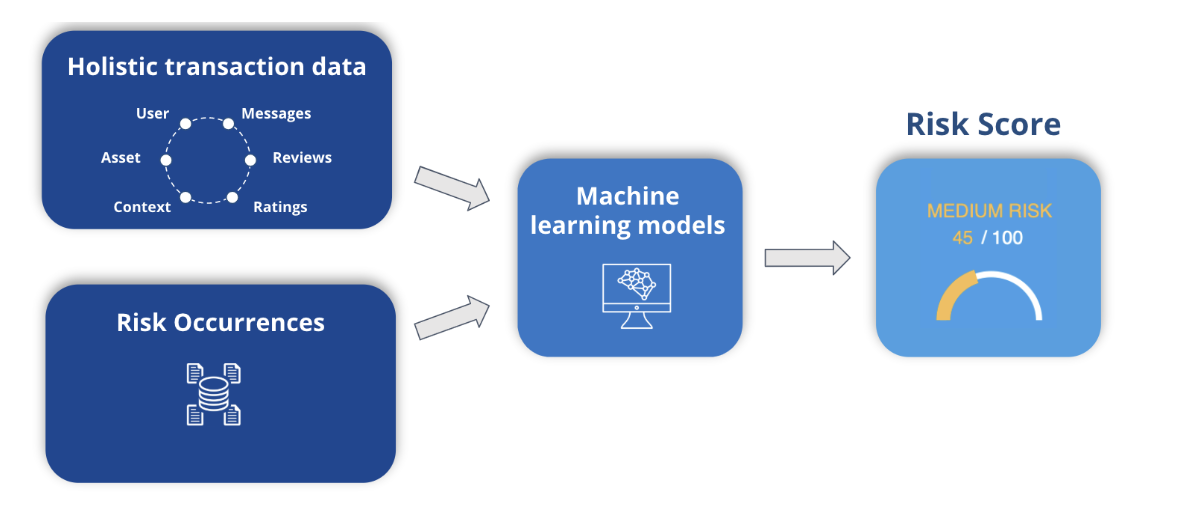
With this framework, a good ability to model the risk will deliver risk scores that are highly predictive of the likelihood of a risk occurrence. An aoutstanding performance depends on every step of the process: from your internal data collection, to the evaluation of the different 3rd party data sources, the feature engineering to prepare the variables, properly training and calibrating machine learning models and bringing everything to production.
Examples of Vehicle Sharing Marketplaces addressing their biggest risk (Physical Damage) with this framework
Below is a performance benchmark we observed while predicting physical damage losses at vehicle sharing marketplaces. With the custom scores created, we were able to consistently identify a few transactions (representing ~3% of total revenue) that were up to 10X more risky than the low risk transactions (accounting for 24% of the revenue).
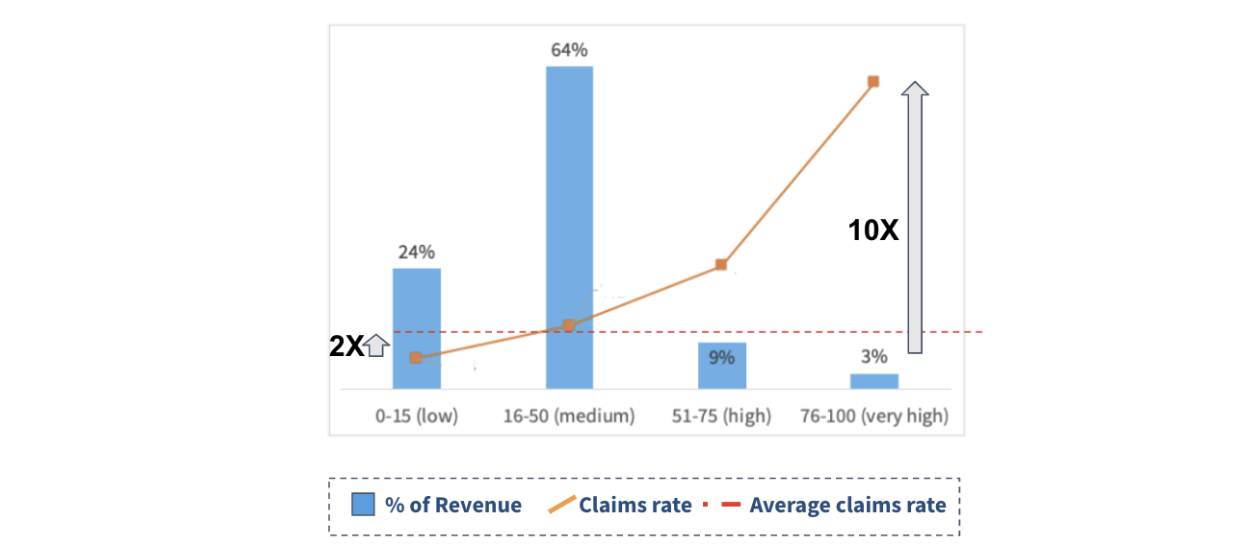
The immediate question that comes after identifying transactions that are up to 10X more risky is how much of the total risk they represent. In this example, the long tail of transactions contains up to 25% of the risk. With this segmentation capability, 2 practical actions can be taken, at the transaction level:
- filtering out the very risky transactions
- dynamically pricing the insurance based on risk level
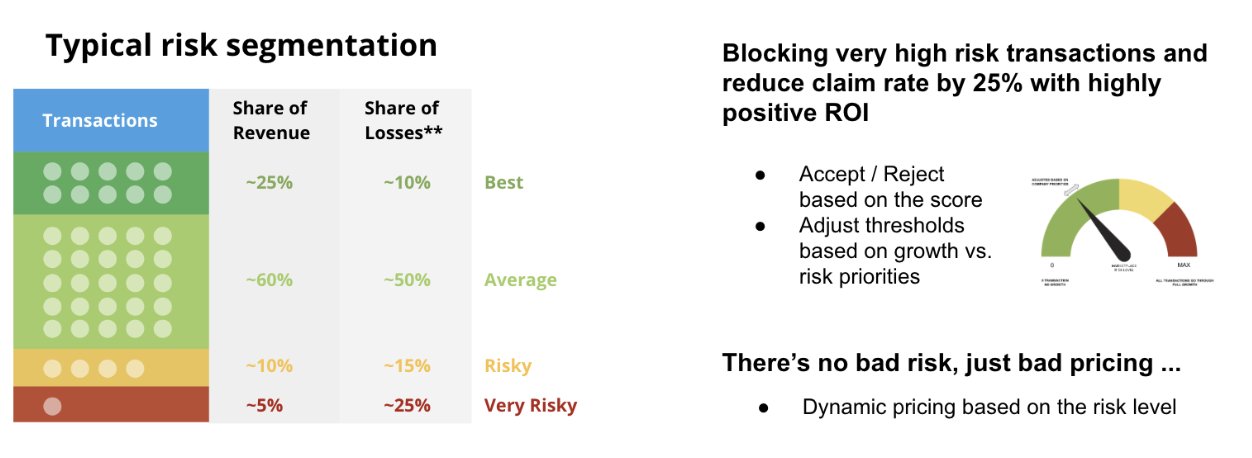
With the strength of this segmentation and the characteristics of the marketplace (average claim, net margin per transaction), the option to apply risk selection to filter out the very high risk transaction is almost neutral on transaction volume but highly impactful on the losses incurred by the marketplace. In that case, that is a very ROI positive process that significantly improves the profitability of the marketplace overall.
By pricing dynamically the risk component of the transaction (in that case the physical damage protection package), the marketplace is providing a fairer pricing based on the use case and encouraging lower risk transactions. To fully grasp the impact on the P&L, a proper A/B test needs to be run. In addition to the calculation of the expected loss (also called raw premium) the dynamic price should also take into account the elasticity of the demand, which requires building additional models, in order to maximize the profit of the insurance program.
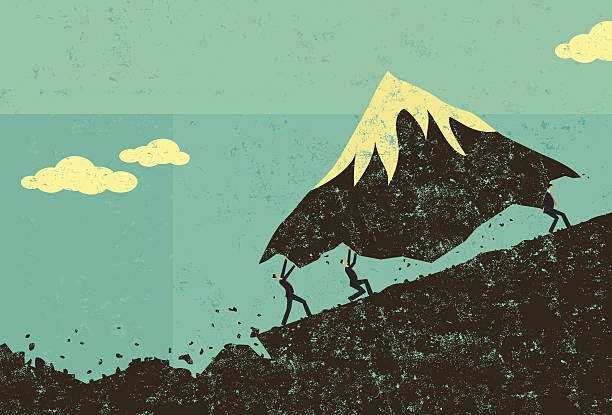
Interested in learning more about this framework and how it can help you manage your risk and your insurance products better? Reach out to Tint today!